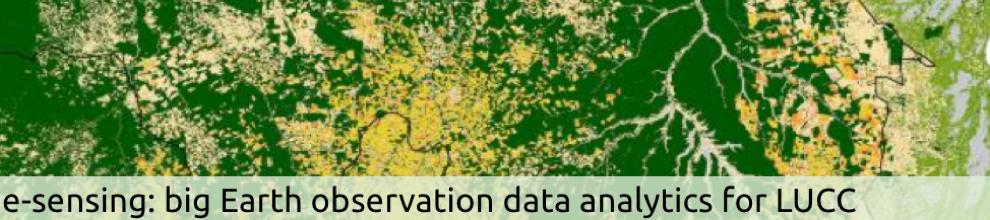
e-sensing Software
Github site
Our github site has a number of useful tools for big Earth Observation data analysis, including the packages described below.
R package for Satellite Image Time Series
The sits package (Satellite Image Time Series) includes data retrieval from a WTSS (web time series service), different visualization methods for image time series, smoothing methods for noisy time series, and different clustering methods including dendrograms and SOM. It matches noiseless patterns with noisy time series using the TWDTW method for shape recognition and provides machine learning methods for time series classification. To our knowledge, sits is the first R package that provides a unified support for machine learning methods for satellite image time series. The package includes an advanced method for data clustering based on self-organized maps (SOM), and also links to a recent advances on spatiotemporal interval logic, both described in the next section.
The methods available for machine learning in sits include:
- Support vector machine (svm): a classifier that uses linear and non-linear mapping of the input vectors into high-dimensional spaces, building hyperplanes that allow distinguishing between the data classes.
- Random Forest (rfor): ensemble learning method for classification, that works by building a multitude of decision trees at training time.
- Linear Discriminant Analysis (lda): a method that finds a linear combination of features that characterizes or separates the desired classes.
- Quadratic Discriminant Analysis (qda): A methods that separate measurements of two or more classes of objects by a quadric surface.
- Deep learning using multilayer perceptrons (dl-mlp): A method that uses a cascade of multiple layers of neural networks with nonlinear processing units.
The "sits" package is available at the e-sensing gitub site .
R package for Time-Weighted Dynamical Time Warping (TWDTW)
We have produced dtwSat , an R package that implements the time-weigthed dynamic time warping algorithm. The method improves on the well-known dynamical time warping (DTW) method for time series data mining. We introduce a temporal constraint that accounts for the agricultural calendar. The resulting algorithm achives better results than previous research that has used DTW for remote sensing image classification. The package is fully described in a paper on the Journal of Statistical software .
Spatiotemporal calculus for reasoning about land use change dynamics
The e-sensing project developed a spatiotemporal calculus for reasoning about land change dynamics. When analyzing Earth observation data, scientists are particularly interested in land use trajectories, which are paths from one land use into another. Typical questions researchers would like to ask are: Which forest areas were degraded from 2000 to 2017? When did new agricultural systems such as double-cropping were introduced in the regions? Which area changed for pasture to croplands in the past decade?
To allow researchers to reason about these and similar change, we propose a land use change calculus (LUC Calculus), whose R implementation is available at the e-sensing gitub site .
Web time series services
The Web Time Series Service is an important result of the e-sensing project. WTSS is a lightweight web service. The client allows Earth observation users to obtain time series from data sets available in a Web Time Series Server. The functions include: (a) listing the data sets available in the server
The WTSS client for R is available in the CRAN repository.